With the surge in popularity of ChatGPT and the flourishing of AGI, Nvidia is developing at an unprecedented pace, which has not only led to the prosperity of GPUs but also kept the heat on High Bandwidth Memory (HBM), playing a crucial role.
Following Micron and SK Hynix's recent statements that their HBM production capacity for this year has been fully booked, Micron and Samsung have also recently introduced new HBM products, hoping to secure a share in this booming market. Among them, the former has brought products that will be used in Nvidia's GH200, and also stated that it will bring a 36 GB 12-Hi HBM3E product in March 2024, while the latter has stated that the company's released HBM3E 12H has increased performance and capacity by more than 50%.
It can be seen that the competition in HBM is becoming increasingly fierce, and HBM has also become the key to determining the fate of AI chips. This is why Timothy Prickett Morgan believes that whoever controls HBM controls AI training.
Here is the full text shared by Timothy Prickett Morgan:
What is the most important factor driving the development of Nvidia's data center GPU accelerators in 2024?
Is it the upcoming "Blackwell" B100 architecture? Are we sure that this architecture will provide a leap in performance compared to the current "Hopper" H100 and its fat memory brother H200? No.
Advertisement
Is it the company's ability to get back millions of H100 and B100 GPU chips from its foundry partner TSMC? No, it is not.
Is it Nvidia's AI Enterprise software stack and its CUDA programming model and hundreds of libraries? In fact, at least some of this software (if not all) is the de facto standard for AI training and inference. However, it is not.
While all of these are undoubtedly huge advantages and are the advantages that many competitors are focusing on, the most important factor driving Nvidia's business in 2024 is related to money. Specifically: Nvidia ended the 2024 fiscal year in January with cash and bank investments slightly below $26 billion. If this fiscal year proceeds as expected, revenue will exceed $100 billion, with more than 50% of it reflected in net profit. Even after paying taxes, a huge R&D business, and the company's normal operating expenses, it will add about $50 billion to its treasury.
You can do a lot with $75 billion or more, one of which is not having to worry too much about the huge amount of money needed to purchase HBM stack DRAM memory for data center-level GPUs. This memory is becoming faster, denser (in terms of gigabits per chip), and fatter (FAT, in terms of megabit bandwidth and gigabyte capacity) at a fairly good rate, but its improvement speed has not kept up with the needs of AI accelerators.As Micron Technology joins the ranks of suppliers for SK Hynix and Samsung, the supply of High Bandwidth Memory (HBM) has improved, along with its feed rate and speed. We strongly suspect that the supply will not meet the demand, and the price of HBM memory will continue to rise, driven in part by the price of GPU accelerators that HBM influences to a certain extent.
AMD has $5.78 billion in cash and investments, and does not have a lot of idle funds. Although Intel's bank deposits are slightly higher than $25 billion, it must build a foundry, which is indeed very expensive (currently popular in sequence, it costs between $15 billion to $20 billion each time). Therefore, it also cannot squander on HBM memory.
Another factor favorable to Nvidia's GPU accelerator business is that during the GenAI boom, customers are willing to pay almost any price for hundreds, thousands, or even tens of thousands of data center GPUs. We believe that the price of the original "Hopper" H100 GPU announced in March 2022, especially in the SXM configuration, for a single H100 with 80 GB of HBM3 memory and a speed of 3.35 TB/s, is over $30,000. We do not know the cost of the H100 with 96 GB of memory and a speed of 3.9 TB/s, but we can speculate on Nvidia's charges for the H200 device with 141 GB of HBM3E memory, running at a speed of 4.8 TB/s. The H200 is based on the exact same "Hopper" GPU as the H100, increasing the memory capacity by 76.3% and the memory bandwidth by 43.3%, and improving the performance of the H100 chip by 1.6 to 1.9 times. Considering that the additional capacity means fewer GPUs are needed and less power is consumed to train a given model for a static dataset, we believe Nvidia can easily charge 1.6 to 1.9 times more for the H200 compared to the original H100.
Golden Rule: Those who have the gold make the rules.
We are not saying that this will happen when the H200 starts shipping in the second quarter. (We believe Nvidia talks about calendar quarters in addition to financial data.) We are just saying that such a move is logical. It largely depends on how much AMD charges for the "Antares" Instinct MI300X GPU accelerator, which has 192 GB of HBM3 running at 5.2 TB/s. The MI300X has more raw floating-point and integer capabilities, with 36.2% more HBM capacity than Nvidia's H200, and 10.4% more bandwidth than the H200.
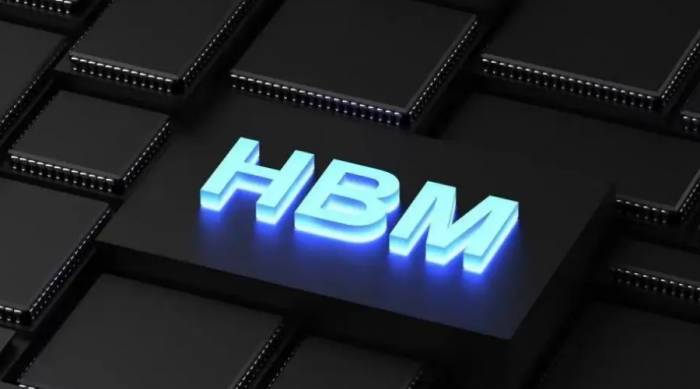
You can bet with Elon Musk's last dollar that AMD is not in the mood to do anything other than charge as much as possible for the MI300X, and there are even suggestions that the company is working to upgrade to fatter, faster HBM3E memory to maintain competition with Nvidia. The MI300 uses HBM3 with eight-high DRAM stacks, and the memory controller in the MI300 has signal and bandwidth capacity that can be replaced with a faster twelve-high stack HBM3E. This means a 50% increase in capacity and a possible 25% increase in bandwidth. That is to say, each MI300X has 288 GB of HBM3E capacity and a bandwidth of 6.5 TB/s.
It is speculated that such a well-designed MI350X chip (which we might call it) performs a considerable amount of actual workload, or even more, in its peak failure times, just as happened when Nvidia jumped from the H100 to the H200.
It is against this backdrop that we want to talk about what is happening in the HBM field. We will start with SK Hynix, which has demonstrated a 16-chip high HBM3E stack, each providing 48 GB of capacity and 1.25 TB/s of bandwidth. The MI300X is equipped with 8 memory controllers, enabling 384 GB of memory and 9.6 TB/s bandwidth.
With these numbers, you no longer need to use the CPU as an extended memory controller to handle a large amount of workloads...
We have not yet seen the introduction of SK Hynix's sixteen-high HBM3E memory, nor do we know when it will be available. In August last year, SK Hynix demonstrated the fifth-generation HBM memory and the first-generation HBM3E memory, which is said to provide a bandwidth of 1.15 TB/s per stack. As shown in the HBM roadmap created by Trendforce below, our expectation is to provide 24 GB and 36 GB capacities, which means eight-high stacks and twelve-high stacks.In August last year, Nvidia was clearly set to become a major customer for these chips, and there were rumors that SK Hynix's 24 GB HBM3E memory would be used for the upcoming "Blackwell" B100 GPU accelerator. If so, then the six memory controllers on the Blackwell GPU chiplets would produce a capacity of 144 GB, and if the B100 package is expected to have two GPU chiplets, it would mean a maximum capacity of 288 GB with a bandwidth of 13.8 TB/s. It is difficult to say what the yield rate is, and it may only be available at 5/6. It is also possible - but we hope not - that the B100 does not look like a GPU, but rather two GPUs of the system software (just like the two chipset AMD "Arcturus" MI250X does, unlike the MI300X, which has 8 smaller GPU chiplets that add up to more charm and look like a GPU to the system software). We will see what happens there.
Micron Technology entered the HBM field later, but given the shortage of supply and strong demand, the company is undoubtedly the most popular in this field. The company said today that it is starting to produce its first HBM3E memory, which is an eight-high stack with a capacity of 24 GB, and added that this memory is part of the H200 GPU. We introduced the Micron HBM3E variant last July, which has a pin speed of 9.2 Gb/s and provides 1.2 TB/s of memory per stack. Micron also claims that its HBM3E memory consumes 30% less than "competitive products," presumably referring to strict HBM3E comparisons.
Micron also said that it has started sampling its 12-high 36 GB HBM3E variant, which will run at speeds exceeding 1.2 TB/s. Micron did not disclose how much faster than 1.2 TB/s.
Later today, Samsung launched the twelve-high stack HBM3E, which is also its fifth-generation product, codenamed "Shinebolt."
Shinebolt replaces the "Icebolt" HBM3 memory launched last year. Icebolt stacked DRAM memory provides a bandwidth of 819 GB/s for a twelve-layer stack with a capacity of 24 GB. Shinebolt HBM3E provides a bandwidth of 1.25 TB/s in a 36 GB stack, just like the SK Hynix HBM3E twelve-high stack.
Samsung added in the announcement: "When used for AI applications, it is expected that the average speed of AI training will increase by 34% compared to using HBM3 8H, while the number of concurrent users for inference services can be increased by 34%." Expanded by more than 11.5 times." Samsung noted that this is based on internal simulations, not actual AI benchmarks.
Samsung's Shinebolt HBM3E 12H is now available for sampling and is expected to be fully produced by the end of June.These 12-high and 16-high HBM3E stacks are almost all we have until the release of HBM4 in 2026. People might hope for HBM4 to appear in 2025, and there is undoubtedly pressure to push the roadmap upgrade, but it seems unlikely. It is speculated that the memory interface of HBM4 will double to 2,048 bits. HBM1 to HBM3E used a 1,024-bit memory interface, and the signal transmission speed has increased from 1 Gb/s to 9.2 Gb/s compared to the initial HBM memory designed by AMD and SK Hynix and delivered in 2013. Doubling the interface will allow for twice the speed. A large amount of memory is required to suspend the interface and provide a given amount of bandwidth at half the clock speed, and as the clock speed increases again, the bandwidth will gradually increase. Alternatively, they were launched at a speed of 9.2 Gb/s per pin from the start, and we just have to pay the price in watts.
Micron's roadmap indicates that HBM4 will offer capacities of 36 GB and 64 GB, with drive speeds of 1.5 TB/s to 2 TB/s, so it seems to be a mix of wide speed and slow, wide speed and faster, but it will not fully meet the demand at the time of release. Speaking of bandwidth. It seems that doubling the width can almost double the capacity and bandwidth. HBM4 is expected to have a sixteen-layer DRAM stack, and that's it.
In another dream world of 2026, HBM4 will have a 2,048-bit interface, similar to a 11.6 Gb/s signal transmission on the pins, with 24 high DRAM stacks, with DRAM memory at a density of 33.3% (4 GB instead of 3 GB), so the speed per stack is about 3.15 TB/s, and the capacity per stack is about 96 GB. Oh, let's go crazy. Assuming a GPU complex has a dozen small chips, each with its own HBM4 memory controller. This would provide an aggregate memory bandwidth of 37.8 TB/s for each GPU device, and a capacity of 1,152 GB per device.
From this perspective, according to Nvidia, a 175-billion-parameter GPT-3 model requires 175 GB of capacity for inference, so the theoretical GPU's memory size we are discussing can handle about 1.15 trillion parameter inferences. For GPT-3 training, 2.5 TB of memory is needed to load the data corpus. If your Hoppers have 80 GB of HBM3 memory, you would need 32 Hoppers to do the job. But our capacity of 32 devices will increase by 14.4 times, so it can load a correspondingly larger amount of data. We assume that the bandwidth on our device is also 11.3 times higher.
Note that we did not mention the failure of these dozen GPU small chips? In most cases, it is very tricky to run anything at more than 80% utilization, especially when it may perform different operations with different precisions. What we want is to restore the trigger-to-bit/second ratio to normal. We want to build a 12-cylinder engine with enough injectors to actually feed the beast.
Our guess is that the 80 GB HBM3 memory of H100 is about one-third of the ideal value, and the bandwidth is also about one-third of the ideal value. This is a way to maximize GPU chip sales and revenue, as Nvidia has clearly proven, but it is not the way to build a balanced computing engine - just like Intel put half of the DRAM memory controllers on its X86 chips and sold them all to us - two sockets with middle bin parts have always been the correct answer for general computing in data centers. We also need more memory capacity and bandwidth.
So, if the bandwidth is increased by 11.3 times with this conceptual Beast GPU accelerator, the computation may only be increased by 4 times compared to the original H100. On the tensor core, H100 is rated at 67 teraflops in FP64 precision and 1.98 petaflops in FP8 precision (without sparsity). Therefore, this TP100 GPU complex is rated at 268 teraflops in FP64 and 7.92 petaflops in FP8, and the performance of each GPU small chip will be one-third of the H100 chip performance, and may be one-fourth to one-fifth of its size, depending on the process technology used. Assuming it is TSMC 2N or Intel 14A compared to the TSMC 4N used on the real H100. After all, this is 2026 we are talking about.
This is the kind of beast we want to write, if we have $26 billion in the bank and a prospect of more than $50 billion in the future, this is what we would do. But a lot of HBM memory and computing engines are stuffed with it.
It is difficult to say how much this will cost. You can't call Fry's Electronics to ask what the market price of HBM4 memory is in 2026. On the one hand, Fry's is already dead. On the other hand, we can't even understand well now how much GPU and other matrix engine manufacturers pay for HBM2e, HBM3, and HBM3e memory. Everyone knows (or thinks they know) that HBM memory and any intermediary layer used to link memory to devices are the two main costs of modern artificial intelligence training and inference engines. (Of course, except for those who mix on-chip SRAM and ordinary DRAM.)In the market, the largest, thickest, and fastest 256 GB DDR5 memory modules for servers running at 4.8 GHz are priced at about $18,000, approximately $70 per GB. However, thinner modules that are only scalable up to 32 GB cost just $35 per GB. Thus, the price for HBM2e is about $110 per GB, "more than 3 times" as shown in the Nvidia chart above. A 96 GB unit is priced at around $10,600. It is difficult to say how much the advancements to HBM3 and HBM3E might be worth in terms of the "market price" of the device, but if the upgrade to HBM3 only increases the price by 25%, then the market price for the H100 with 80 GB capacity would be about $30,000, with the cost of HBM3 at $8,800. Switching to 96 GB HBM3E could raise the memory cost to a "market price" of $16,500, as the technological cost increases by another 25%, and the additional 16 GB of memory and the market price for the H100 96 GB should be about $37,700.
It would be interesting to hear rumors about the price of the H200 with a capacity of 141 GB (for some reason, not 144 GB). But if this memory price stratification holds—realizing that these are wild estimates—then 141 GB of HBM3E itself would be worth about $25,000. But at such a price, the "market price" for the H200 would be about $41,000. (Note: This is not what we think Nvidia pays for HBM3 and HBM3E memory—it's not the bill of materials cost—but rather the price allocated to the end user.)
We believe the increase will not exceed about 25%, as upgrading the memory to HBM3 and then to HBM3E will push the memory price higher, above the rumored Nvidia GPU prices in the market.
Remember, this is just a thought experiment to illustrate how HBM memory pricing controls the number of GPUs that Nvidia and AMD can put into the field, not the other way around. The memory tail is wagging the GPU dog. The memory capacity and bandwidth are becoming increasingly intertwined with the H200, and if Nvidia only charges a nominal fee for the additional memory and its extra speed, not only would the actual efficiency of the device increase, but so would the cost-performance ratio. But if Nvidia just prices these more powerful H100 and H200 in such a way that the performance gains and memory gains balance out, then less money would be spent, and more money would be required.
To be honest, we don't know what Nvidia will do, nor do we know what AMD will do after the MI300 gets an HBM3E upgrade. Now that Micron has entered the field of HBM suppliers, increasing by 50%, and SK Hynix and Samsung have doubled their production, it's a big number, but compared to the GPU and the demand for GPUs, the HBM memory in the market has only increased by 3 times. Their memory is larger, arguably more than 3 times. This is not an environment where prices can be lowered. In this environment, people would raise the prices of more advanced computing engines and their memory and continue to scale down HBM memory as thin as possible.
This is why as long as the Nvidia platform continues to be the preferred choice, those who can afford to pay high prices for HBM memory (i.e., Nvidia co-founder and CEO Jensen Huang) can set the pace and price of artificial intelligence training.
In other words, for GPUs and HBM, they are both facing a do-or-die situation.
Post a comment